OnlyPrompts介绍
OnlyPrompts是一款收录了超过150000个Prompts的AI平台,用户可以查找Prompts并解决超过30000个任务。
OnlyPrompts提供免费版本和付费版本,免费版本有300个Credits,每次使用不同的AI模型会消耗不同个数的Credits。付费版本可以购买更多Credits。
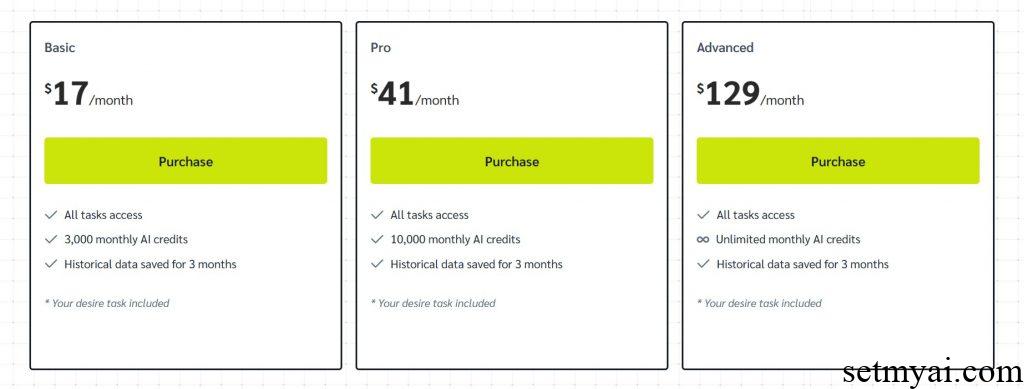
如何使用OnlyPrompts
我们使用OnlyPrompts查找有关人工智能算法的Prompts。OnlyPrompts已经将不同的Prompts划分为各种类别,并提供筛选功能。我们可以从部门、角色、行业和兴趣的角度进行筛选,也可以从页面上方的搜索栏中输入关键词筛选。OnlyPrompts为每一个筛选维度提供了大量的选择,基本可以覆盖用户日常的搜索需要。
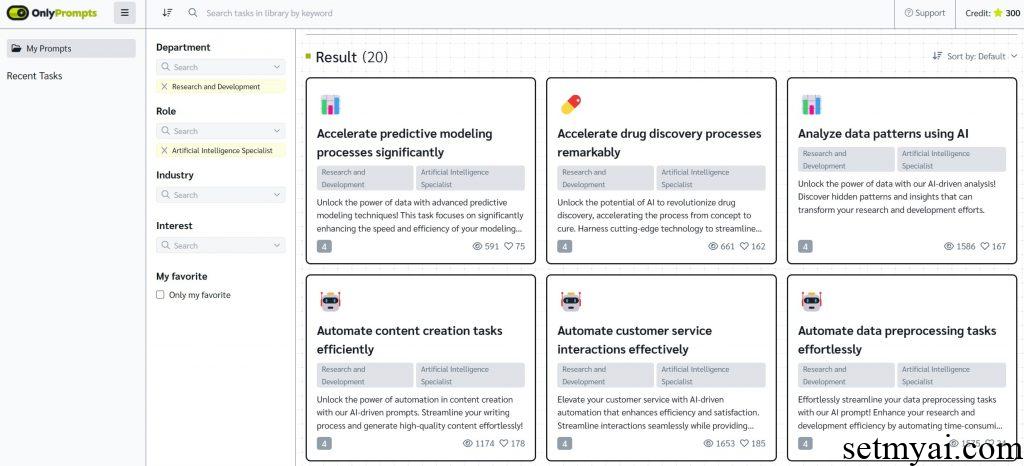
在我们设置筛选后,OnlyPrompts提供了有关人工智能研究的一些Prompts。我们点击Develop machine learning algorithms efficiently,进入具体的页面。我们可以从页面中修改关键词,并使用AI生成结果。OnlyPrompts提供超过十种不同的AI模型,以及数十种语言。当我们完成修改后,点击Run,即可查看AI提供的结果。
As an Artificial Intelligence Specialist in the Research and Development department, you will assist me in developing efficient machine learning algorithms. Begin by outlining the specific algorithm type: ‘[specific algorithm type]’ that best fits our needs. Next, identify the target application domain: ‘[target application domain]’ where this algorithm will be implemented. Specify the desired output metrics: ‘[desired output metrics]’ that will define the algorithm’s success. Additionally, provide insights into the current challenges: ‘[current challenges]’ we are facing in this process. Finally, list the available resources: ‘[available resources]’ that can be utilized for algorithm development. Ensure that your response is structured logically and clearly, highlighting each step comprehensively.
Certainly! Let’s outline the development of an efficient machine learning algorithm step by step.
### 1. Specific Algorithm Type:
**Type: Random Forest Classifier**
The Random Forest Classifier is an ensemble learning method that constructs multiple decision trees during training and outputs the mode of the classes for classification problems. It is well-suited for handling a mix of numerical and categorical data and is robust against overfitting, making it a good choice for many real-world applications.
### 2. Target Application Domain:
**Domain: Healthcare Analytics**
In the healthcare analytics domain, the algorithm can be applied to predict patient outcomes, such as disease diagnosis, treatment effectiveness, or predicting readmission rates. This domain benefits from the ability to handle complex datasets with various features, including patient demographics, clinical data, and historical health records.
### 3. Desired Output Metrics:
**Metrics:**
– **Accuracy:** The percentage of correctly predicted instances over the total instances.
– **Precision:** The ratio of true positive predictions to the total predicted positives, indicating the quality of the positive predictions.
– **Recall (Sensitivity):** The ratio of true positive predictions to the actual positives, measuring the algorithm’s ability to identify all relevant instances.
– **F1 Score:** The harmonic mean of precision and recall, providing a balance between the two metrics.
– **AUC-ROC Curve:** The area under the Receiver Operating Characteristic curve, which illustrates the trade-off between sensitivity and specificity.
### 4. Current Challenges:
**Challenges:**
– **Data Quality:** Incomplete or noisy data can significantly impact the performance of the Random Forest algorithm. Preprocessing and data cleaning are essential but can be resource-intensive.
– **Feature Selection:** The presence of irrelevant or redundant features can lead to overfitting. Identifying the most important features requires careful analysis and potentially additional techniques.
– **Imbalanced Data:** In healthcare, certain outcomes may be rare, leading to imbalanced datasets. This can skew the results and affect the accuracy of the model. Techniques like SMOTE (Synthetic Minority Over-sampling Technique) may be needed to address this.
– **Interpretability:** While Random Forests provide good performance, they can be less interpretable than simpler models, which may be a concern in healthcare settings where understanding the decision-making process is crucial.
### 5. Available Resources:
**Resources:**
– **Data Sources:** Access to electronic health records (EHRs), clinical databases, and public health datasets for training and testing the model.
– **Computational Resources:** High-performance computing clusters or cloud-based platforms (e.g., AWS, Google Cloud) to handle the computational demands of training the model, especially with large datasets.
– **Software Libraries:** Utilization of machine learning libraries such as Scikit-learn (Python), TensorFlow, or PyTorch for model implementation and evaluation.
– **Expertise:** Collaboration with healthcare professionals and data scientists to ensure that the model is grounded in clinical relevance and addresses real-world problems effectively.
By following this structured approach, we can develop an efficient Random Forest algorithm tailored to the healthcare analytics domain, ultimately improving patient outcomes and operational efficiencies.
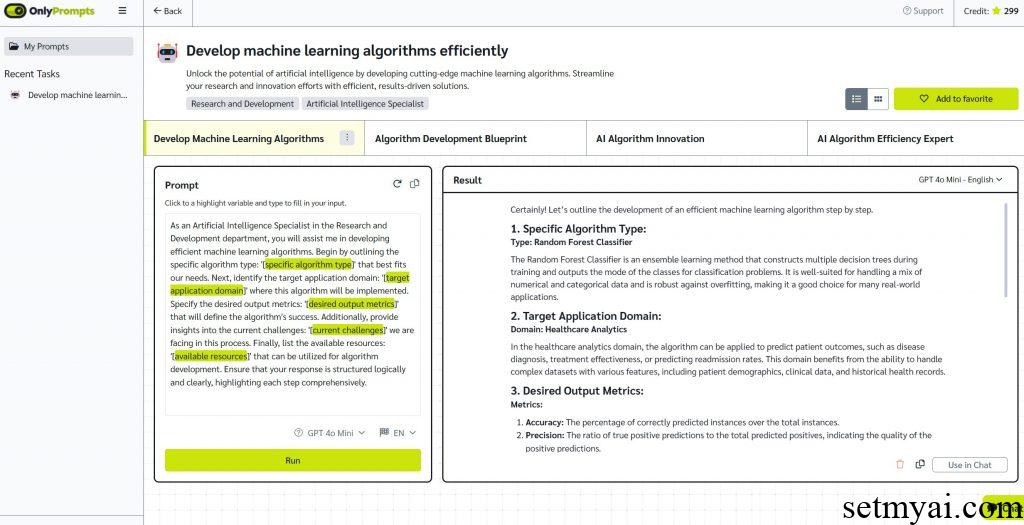
总结
作为一款收录了超过150000个Prompts的AI平台,OnlyPrompts能够为用户提供高质量的Prompts。SetMyAI推荐对撰写Prompts感兴趣的用户使用这一工具。
网址: